Is it possible that after all these years of evolution in computer-aided design and simulation, we’ve still only scratched the surface of visual computing’s potential? Stepping back and thinking about the typical projects which rely on CAD — buildings, physical infrastructure, and personal and industrial products of all kinds — our focus has been primarily or exclusively focused on those projects’ infancy. We sketch, draw, simulate, visualize, and iteratively refine until we’ve got a virtual model ready for physical implementation. And hopefully, but sadly not always, the virtual can be converted to the physical in an automated flow, with minimal manual rework. And, then, we’re basically done. Sure, models will often be leveraged for subsequent product refinements or even debugging, but essentially the project is archived and we move on to the next design.
But while the job of the designer and creator is done, the actual life of the product is just starting. Chances are that its lifetime will last far longer than the design and development did. So why not leverage all that design insight to optimize the product’s physical life as much as its virtual creation? That’s the premise behind one of the emerging uses of the digital twin and the metaverse. It’s a concept introduced in previous columns, and one now being adopted by some of the biggest names in automotive, architecture, and construction industries.
NVIDIA promoting Omniverse as a key enabler for digital twin applications. Image source: NVIDIA.
From Creation to the End of its Physical Life: The Digital Twin as an End-to-End Computing Environment
At first blush, we could look at the metaverse and a digital twin as simply a more physically accurate and comprehensive environment for more traditional CAD objectives, one that imparts as much detail in a virtual product’s world as it does in the product itself. And, yes, the fuller scope of that environment can yield feedback and cues in physical simulation, operations, and lighting that might otherwise be missed with a model focused only on the part with minimal reality in its surrounding world.
Thinking about a more conventional lighting simulation (although conventional shouldn’t be interpreted as trivial) as for example rendering complex outdoor scenes like the tree-lined street below. Factor in many iterations in a daybreak-to-sunset simulation, and the compute burden becomes enormous, but one well-suited to the cloud-hosted metaverse.
Physically based outdoor lighting with complex scenery using simulation. Image source: NVIDIA.
On an even grander scale, consider Siemens’ use of Omniverse in the design and layout of an off-shore wind farm. Within Omniverse, Siemens ran thousands of CFD simulations to ascertain the best possible guide the placement and orientation of each turbine to maximize generation throughput.
Siemens’ wind farm design showcases the power of design in the metaverse. Image source: Siemens.
The wind farm project highlights the unique power of a cloud-hosted, GPU-powered metaverse versus traditional client-side workstation computation. Where one iteration at full simulation precision would bog down the fastest multi-cored CPU for not just hours but days, simulations in Omniverse instead not only delivers the accuracy of the complete natural environment of wind and weather, it can harness Omniverse-integrated components in machine learning, GPU compute-acceleration, and massive scalable performance available in the cloud.
Machine learning, for example, allows each simulation iteration to run at low-grain scale, then intelligently upscaling to fine-grain detail. And a sea of cloud GPUs can run orders of magnitude faster than a single client CPU. The combination let Siemens turn days-long iterations into minutes, in turn enabling far more iterations and more optimal, precise placement of the turbine array. In fact, Siemens believes the Omniverse-based digital twin of that array is so much more optimal and precise, the wind farm will deliver power for an additional 20,000 more homes than otherwise possible.
Another compelling example of a pre-production use of a digital twin in the metaverse is in commercial construction and operations: the factory floor. It’s an application we’ve covered before, and one with huge potential impact to manufacturing construction and operating costs and schedule. By accurately simulating the entire environment in the Omniverse, manufacturers like BMW can best optimize the layout and flow of a future factory floor, avoiding potentially costly reworks in the future physical realm. And, while that factory floor will be increasingly autonomous, driving by ever-more advanced, intelligent robotics, personnel will be there as well, making the interfaces between human and machine as optimal and safe as possible. In its Omniverse environment, NVIDIA has already ensured that tie-in to robotics with its Isaac Sim functionality.
Similarly, consider architectural design in medical facilities, like scan and operating rooms, where doctors, nurses, and patients need the most efficient and comfortable control and access to myriad tools and equipment. Simulating procedures in the metaverse — quite possibly in combination with virtual and augmented reality — uncovers any subtle operating inefficiencies before committing to physical implementation.
A Digital Twin Aging in Parallel
But businesses like NVIDIA and its clients are now looking to exploit the metaverse well beyond the creation side — CAD’s exclusive, historical focus — and into the subsequent physical operation, encompassing the entire lifecycle.
NVIDIA positioning omniverse as an End-to-End Digital Twin environment: from Virtual Creation to Physical Operation. Image source: NVIDIA.
Beyond Omniverse’s fundamental technology enablers — cloud-hosting, real-time rendering and graphics, improved networking speed and latency — there’s more in play making the post-production digital twin functionality more applicable and valuable. A digital model which did not age, fail, or show fatigue would offer limited insight into the health of its physical twin down the road. The availability of cheap, wireless (mostly) sensors at the edge, in combination with machine learning — both at the edge and in the cloud — provides the link in the feedback loop to update a digital twin that’s an accurate reflection not only on its first day of life but its last as well. Plus, measured data gathered from manual inspection can be fed back to maintain the digital twin as well.
A digital twin’s closed feedback loop makes it viable for computer-assisted operations. Image source: Bentley Systems.
A wealth of sensor data is worthless, though, without the intelligence to interpret it. And that’s where the linchpin to Omniverse’s aptitude for managing digital twins lies: machine learning. Cloud-hosted GPUs (in theory complemented by some inference at the edge) running machine learned inference in parallel provide the insight into how the physical aging translates into potential current and future maintenance as well as potential outright failure. Siemens employed a digital twin of its steam turbine to optimize maintenance and predict possible failures before-hand, saving time and money. In another striking example, Singapore is in the process of creating a digital twin of nearly the country’s entire infrastructure, including water, building, and transportation. Early operation actually flagged a potential water main failure before it happened (though due to logistics and timing, not in time to prevent it).
Tying It All Together in the Metaverse
No, we all haven't somehow missed out on an obvious use of our CAD environments all these years. Focusing as much attention not only to a virtual product’s interaction within a fuller, realistic environment, as well as riding shotgun on that product over its lifecycle in its physical world, are applications that simply weren’t practical until recently.
A mature cloud and networking infrastructure, along with real-time (or at least close) physically based rendering of highly complex environments, have created the baseline capabilities to make it a viable proposition. And, the advent of machine learning has created the means to turn real-world lifecycle data into a digital twin that not shows its age in close approximation to its physical sibling but can be used to optimize maintenance, repair, uptime, and functionality to a degree never before possible.
We’ve already had to complicate the CAD acronym over the years. Personally, for simplicity, I tend to use it to encompass CAM and CAE already, and now perhaps it’s time to extend it further, beyond the uses in design, engineering, and manufacturing to operations, mimicking the physical realm over a product’s complete lifecycle.
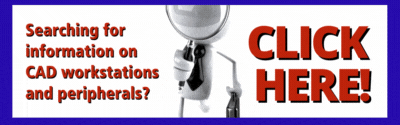
Alex Herrera
With more than 30 years of engineering, marketing, and management experience in the semiconductor industry, Alex Herrera is a consultant focusing on high-performance graphics and workstations. Author of frequent articles covering both the business and technology of graphics, he is also responsible for the Workstation Report series, published by Jon Peddie Research.
View All Articles
Share This Post